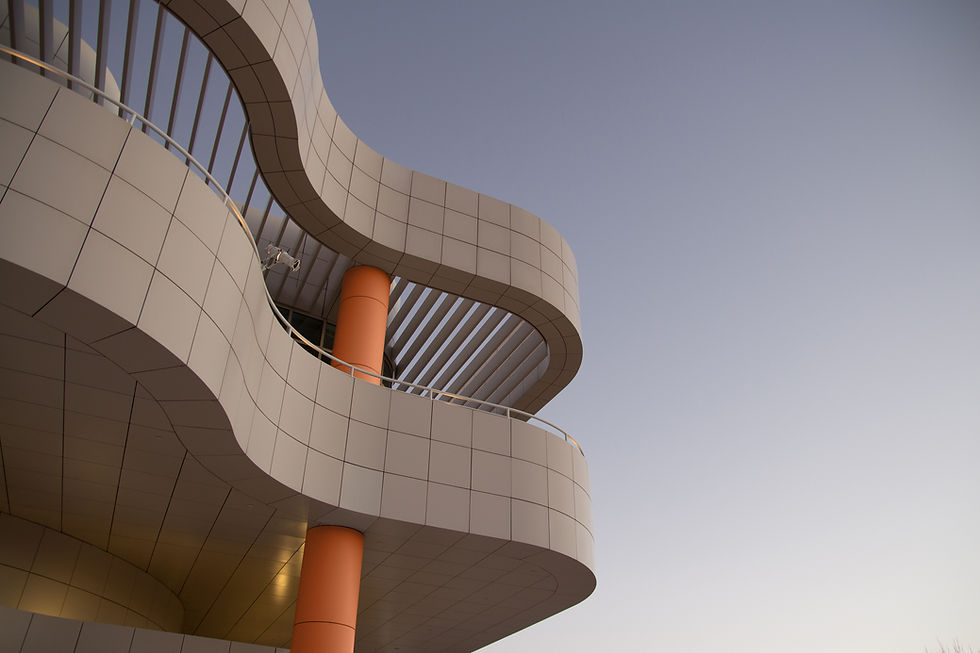
Common Pain Points in Ethical AI and ML Systems
0
0
0
Common challenges in ethical AI and ML Systems include transparency, accountability, bias, interpretability, and ethical implications.
Bias in Data and Algorithms: Bias in data and algorithms poses a complex and pervasive challenge in machine learning. Despite efforts to diversify datasets, machine learning models can perpetuate biases from historical data or societal prejudices. Bias in algorithms often stems from biased training data, leading models to learn and replicate existing biases. This can result in inadvertent discrimination against certain demographics, reinforcing societal prejudices and inequalities. Biased algorithms, especially in high-stakes applications like lending and hiring, can lead to unfair treatment based on attributes like race or gender. This violates fairness principles and can restrict financial access or perpetuate inequality. Addressing bias requires a multi-faceted approach, including dataset curation, algorithm transparency, and ongoing monitoring. Researchers aim to detect and mitigate bias in algorithms, striving for more equitable AI systems that uphold ethical standards and fairness.
Privacy and Consent Challenges: The integration of Artificial Intelligence (AI) systems raises privacy concerns due to reliance on vast datasets. Regulations like GDPR mandate user consent and data anonymization. Businesses face complexities in obtaining consent, anonymizing data, and addressing ethical implications. Prioritizing transparency, accountability, and user empowerment through privacy-by-design principles is crucial for managing privacy challenges in AI technologies.
Transparency and Explainability in Machine Learning: Understanding complex models like deep learning systems is crucial due to their opaque nature, often referred to as "black boxes." Lack of transparency poses challenges in justifying model decisions, especially in regulated sectors like healthcare and finance. The inability to explain ML outputs can lead to skepticism, distrust, and legal issues, hindering fairness, accountability, and compliance. To address this, techniques like model interpretability and algorithmic transparency are being developed to enhance understanding and trust in AI technologies across various industries.
Accountability and Liability in AI: In the realm of artificial intelligence, accountability and liability are crucial. Determining responsibility for errors in AI systems is complex due to the lack of clear guidelines. Accountability gaps can lead to legal and reputational risks. To address this, businesses and policymakers must establish clear roles, oversight processes, and mechanisms for recourse. Proactive measures in accountability and liability help mitigate risks, build trust, and foster an ethical AI ecosystem prioritizing well-being.
Lack of Industry Standards: Although frameworks exist (e.g., ISO 9001 for Quality Management in AI), comprehensive industry standards for ethical AI are still under development. This leaves organizations in uncharted territory when attempting to apply “best practices.”