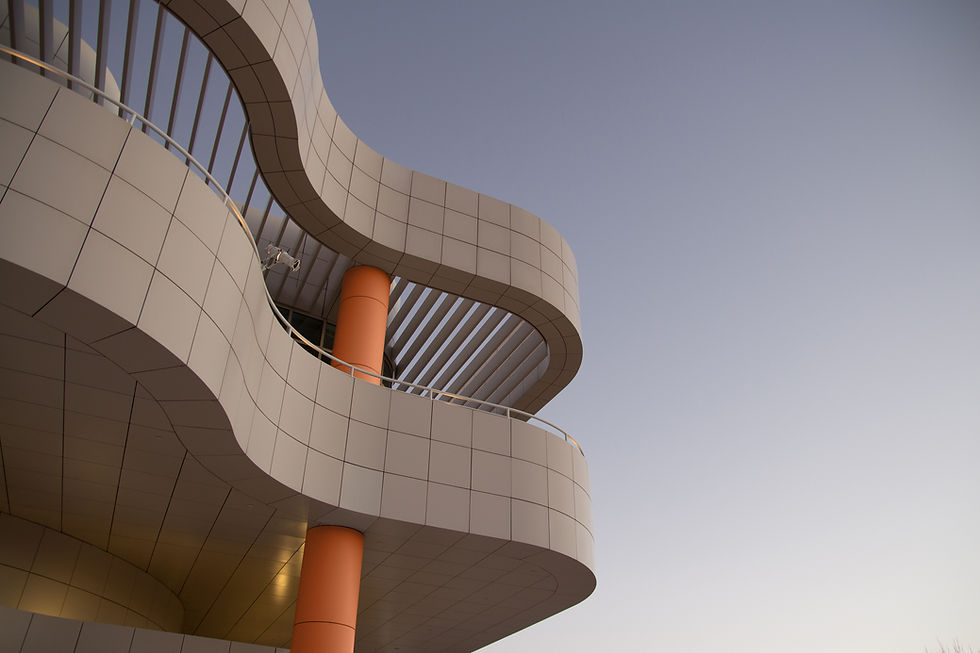
CRISP-DM vs. MLEAP: A Comparative Guide for Machine Learning Projects
0
6
0
CRISP-DM (Cross-Industry Standard Process for Data Mining) provides a methodology for data mining and initial model development, whereas MLEAP (Machine Learning Engineering for Production) emphasizes the engineering, operationalization, and continuous management of machine learning models in production. While CRISP-DM is suited to data exploration and one-off projects, MLEAP is essential for production-level applications that require ongoing monitoring, updates, and scalability.
Below is a comparative table highlighting the similarities and differences between CRISP-DM and MLEAP :
Aspect | CRISP-DM | MLEAP |
Purpose | Provides a structured approach to data mining and analytics projects. | Focuses on engineering and deploying ML models for production. |
Primary Objective | Create an accurate model from data to gain insights or predictions. | Build reliable, scalable, and maintainable ML systems in production. |
Stages/Phases | 1. Business Understanding 2. Data Understanding 3. Data Preparation 4. Modeling 5. Evaluation 6. Deployment | 1. Data Engineering and Pipeline Automation 2. Model Training and Experiment Management 3. Scalable Model Serving 4. Monitoring and Maintenance 5. CI/CD for Machine Learning (MLOps) |
Focus on Business Understanding | Strong: Defines clear business objectives before technical steps. | Often assumed or predefined; focuses on making models deployable. |
Data Engineering | Primarily handled in Data Preparation phase, aimed at getting data ready for analysis. | Central to the process, with robust data pipelines and automated workflows for continuous data flow. |
Model Experimentation | Focuses on trying various algorithms to optimize model performance. | Systematically integrates hyperparameter tuning, model tracking, and management for continuous improvements. |
Deployment | Deployment is the final step, generally less detailed in the process. | Highly emphasized, with CI/CD pipelines and automation for frequent updates and scalability. |
Monitoring and Maintenance | Limited focus on post-deployment monitoring and maintenance. | Core focus, including model drift detection, performance monitoring, and incident alerting. |
Tools and Frameworks | Flexible, often uses data processing and analysis tools, e.g., Python, R, SQL. | Leverages MLOps tools like MLflow, Kubeflow, Docker, and Prometheus for deployment and monitoring. |
Lifecycle Management | Primarily linear, project-focused; model lifecycle management is limited. | Continuous, iterative, production-focused; emphasizes ongoing model performance and updates. |
Reproducibility | Reproducibility supported but less emphasized. | High emphasis on reproducibility using data and model versioning tools. |
Collaboration Requirements | Data scientists, analysts, and domain experts. | Cross-functional: requires data engineers, software engineers, data scientists, and DevOps. |
Use Cases | Ideal for one-time analysis or research-oriented projects in marketing, finance, healthcare, etc. | Essential for long-term, operational ML applications in e-commerce, healthcare, finance, and other industries requiring scalable, reliable models. |